Revolutionizing Business Security: The Impact of Spam Detection Machine Learning
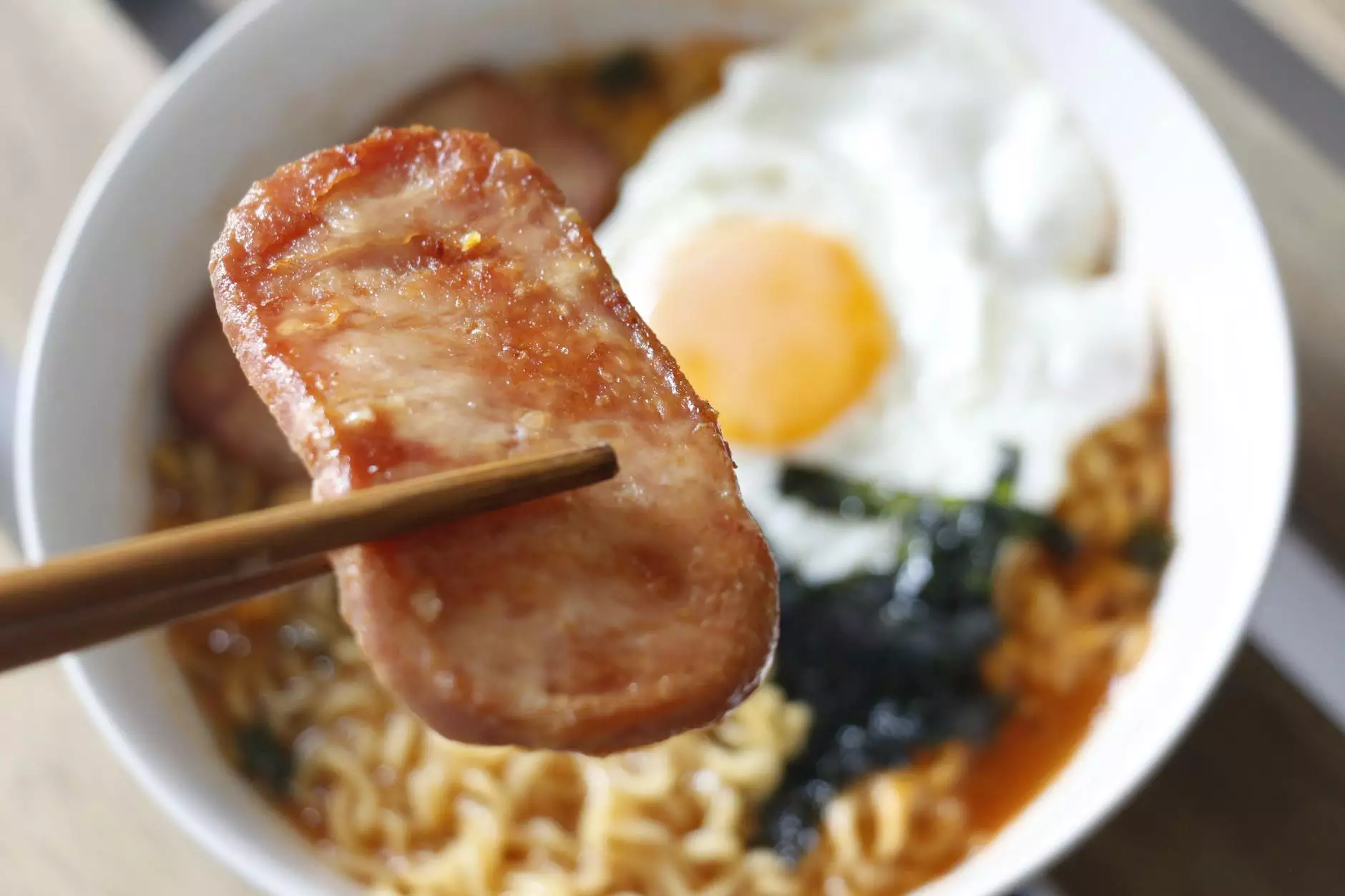
In today's digital age, businesses face an increasing number of threats from spam and malicious attacks. To safeguard their operations, organizations must adopt advanced technologies that enhance their security frameworks. One of the most promising advancements in this field is spam detection machine learning. This innovative approach leverages artificial intelligence (AI) to identify and mitigate spam, ensuring that businesses can effectively protect their vital data and resources.
Understanding Spam Detection: A Brief Overview
Spam detection involves the identification of unsolicited or harmful content that can disrupt business communication and lead to potential damages. Traditionally, spam filters relied on predefined rules and keyword analysis, but as spammers become increasingly sophisticated, these methods have become less effective.
The Role of Machine Learning in Spam Detection
Spam detection machine learning utilizes algorithms that allow computers to learn from data and improve over time without being explicitly programmed. This capability significantly enhances the accuracy of spam detection as the systems can adapt to emerging trends and tactics used by spammers.
How Machine Learning Algorithms Work
Machine learning algorithms analyze vast amounts of data to identify patterns and anomalies. In the context of spam detection, these algorithms process information from thousands of emails, distinguishing between legitimate communications and spam messages. Popular machine learning techniques employed in this area include:
- Supervised Learning: This involves training a model on a labeled dataset where the algorithm learns to classify messages based on examples of both spam and non-spam.
- Unsupervised Learning: Here, the algorithm identifies patterns in the data without prior labeling, uncovering hidden structures that can indicate spam.
- Natural Language Processing (NLP): NLP enables the machine to understand the context and semantics of messages, providing deeper insights into whether an email is spam or not.
Benefits of Implementing Machine Learning in Spam Detection
The integration of spam detection machine learning into business security systems offers numerous advantages, including:
1. Enhanced Detection Rates
Machine learning models can achieve higher accuracy in identifying spam, reducing the risk of missed threats. By learning from new data continuously, these models improve their detection capabilities over time.
2. Reduced False Positives
Traditional spam filters often mistakenly classify legitimate emails as spam. Machine learning algorithms significantly decrease this issue by better understanding the context and intent of messages.
3. Continuous Improvement
As spam techniques evolve, machine learning models can adapt to these changes, learning from new patterns and techniques employed by spammers. This ensures that your business stays one step ahead.
4. Cost Efficiency
Investing in spam detection machine learning solutions can lead to significant cost savings. By reducing the time employees spend managing spam and mitigating potential damages from attacks, businesses can allocate resources more effectively.
Implementing Spam Detection Machine Learning in IT Services
For IT service providers, incorporating machine learning-based spam detection solutions can elevate the security offerings to clients. Here’s how:
Assess Current Security Frameworks
Before integrating new technologies, it’s essential to evaluate existing systems and identify vulnerabilities that spam detection machine learning can address. This assessment can guide the implementation process effectively.
Choosing the Right Tools
There are numerous machine learning tools and platforms available. Companies should select one that aligns with their specific requirements, considering factors such as:
- Scalability: Can the tool handle increasing amounts of data as the business grows?
- Integration: How easily does it integrate with existing IT infrastructure?
- User-friendliness: Is the interface accessible for IT staff to monitor and manage?
Training Staff
Proper training is crucial for maximizing the benefits of any new technology. Ensuring that IT staff understands how to utilize and manage the spam detection machine learning system will lead to better outcomes and a more secure business environment.
Security Systems and Spam Detection
The intersection of security systems and machine learning provides a multi-layered approach to business protection. Here’s how spam detection machine learning plays a role:
Multi-Factor Authentication (MFA)
Integrating spam detection with MFA systems enhances overall security by ensuring that only verified users access sensitive information. Implementing machine learning can fine-tune authentication processes to recognize unusual activity.
Behavioral Analysis
Machine learning can analyze user behavior patterns to detect abnormalities that may indicate a security threat. If a user suddenly starts sending a large volume of emails, the system can flag this activity as potentially suspicious, invoking additional security measures.
Case Studies: Success Stories of Spam Detection Machine Learning
To emphasize the effectiveness of spam detection machine learning, let’s explore some compelling case studies:
1. A Leading Email Service Provider
One of the largest email platforms implemented a machine learning solution that increased spam detection rates from 95% to over 99%. By leveraging user feedback and behavioral analysis, the platform became a leader in email security.
2. An E-commerce Giant
An e-commerce company faced challenges with phishing attempts aimed at its customers. By integrating machine learning algorithms, the company reduced phishing threats by 80%, creating a safer shopping environment for its users.
Future Trends in Spam Detection Machine Learning
The field of spam detection is rapidly advancing, driven by innovations in machine learning. Here are expected trends that businesses should watch for:
1. Improved Natural Language Processing
As NLP continues to evolve, its integration into spam detection systems will become more sophisticated, allowing for better understanding of the nuances in communication styles and contexts.
2. Greater Personalization
Future systems will likely offer personalized spam detection solutions tailored to the unique needs of different businesses, ensuring they receive the protection that specifically addresses their concerns.
3. AI Collaboration
As AI technology advances, spam detection systems will collaborate with other security solutions to create a more cohesive defense against cyber threats, forming an interconnected security ecosystem.
Conclusion: Embracing the Future with Spam Detection Machine Learning
In conclusion, spam detection machine learning represents a significant leap forward in business security. By harnessing the power of machine learning, companies can protect their critical data, enhance productivity, and maintain customer trust. As threats continue to evolve, adopting innovative solutions will be essential for any organization looking to secure their operations in the competitive digital landscape.
As a pioneer in IT services and computer repair as well as security systems, Spambrella is committed to helping businesses leverage cutting-edge technology to combat spam effectively. Embrace the future of business security today by integrating sophisticated spam detection solutions into your operational framework.