Maximizing Efficiency with Image Labeling Tools for Object Detection
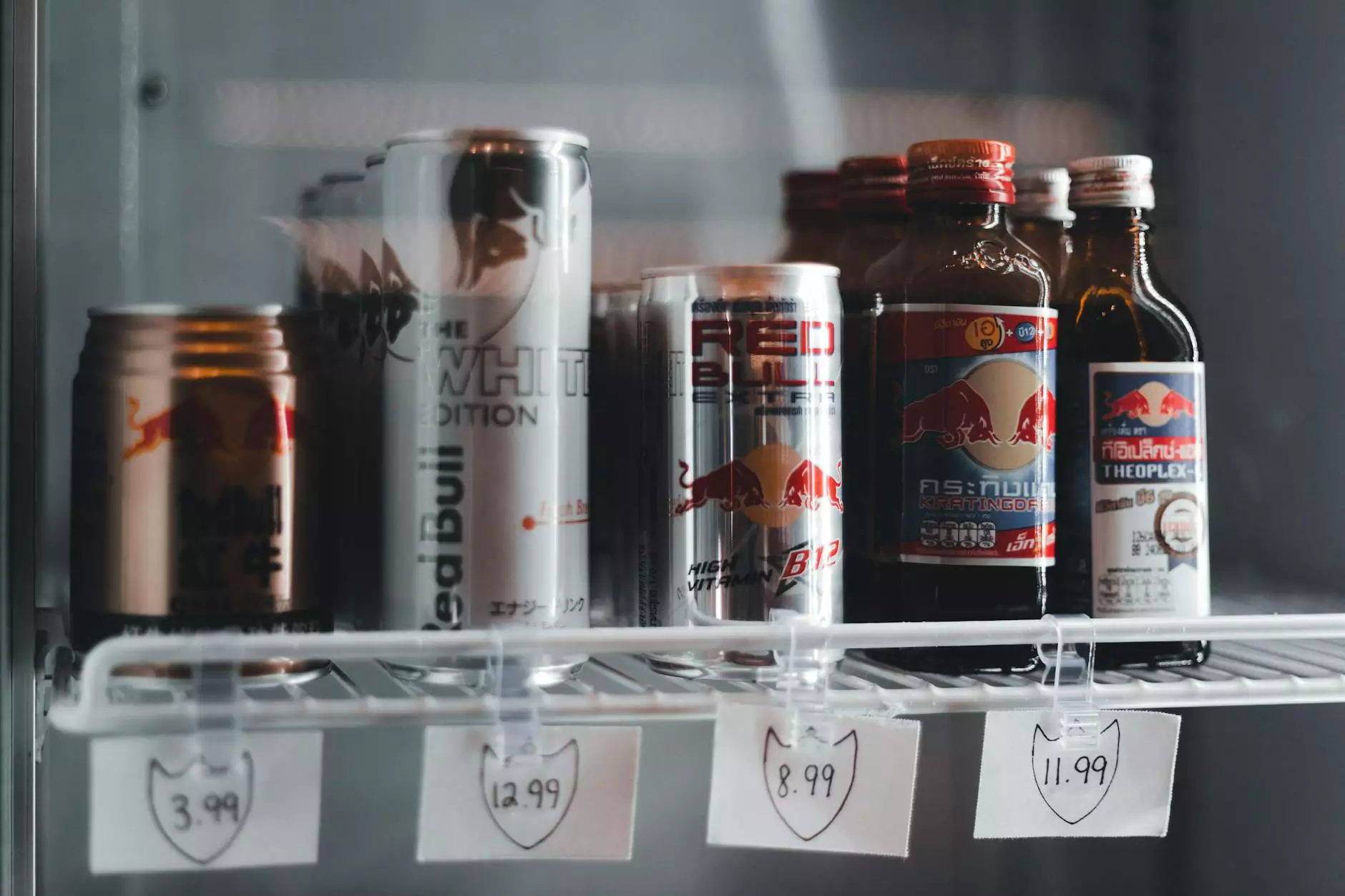
Image labeling tools for object detection have become a cornerstone in numerous industries, especially with the rise of artificial intelligence and machine learning technologies. As businesses seek innovative solutions to enhance their operational efficiency, understanding the importance and application of these tools is critical. In this comprehensive guide, we will explore what image labeling tools are, their significance in object detection, and how they can benefit your business.
What are Image Labeling Tools?
Image labeling tools are software applications designed to assist in the manual or automated labeling of images. These labels can range from simple bounding boxes around objects to complex segmentation masks that identify specific areas of interest within the images. The primary goal of these tools is to provide annotated data that can be utilized to train machine learning models for various applications, including object detection, facial recognition, and scene understanding.
The Importance of Object Detection
Object detection refers to the process of identifying and classifying objects within an image. It has a wide range of applications, including:
- Autonomous Vehicles: Object detection is crucial for self-driving cars to understand their surroundings.
- Surveillance Systems: Enhanced security measures can be achieved through accurate object detection.
- Retail and Inventory Management: Businesses can automate stock management based on object detection capabilities.
- Healthcare: From identifying tumors in imaging scans to monitoring patient activities, object detection plays a vital role.
How Image Labeling Tools Facilitate Object Detection
For an object detection model to perform optimally, it requires high-quality, accurately labeled training data. This is where image labeling tools for object detection come into play:
- Efficient Data Annotation: These tools help streamline the data labeling process, saving both time and resources.
- Enhanced Collaboration: Many image labeling tools support collaborative efforts, allowing multiple users to annotate images simultaneously.
- Variety of Annotations: Users can create bounding boxes, polygons, keypoints, and more, ensuring comprehensive data representation.
- Quality Control: Advanced tools often include features for reviewing and validating annotations, ensuring data quality.
Choosing the Right Image Labeling Tool
Selecting the appropriate image labeling tool for object detection is crucial. Here are some factors to consider:
- User-Friendliness: The interface should be straightforward, enabling users to quickly learn how to annotate effectively.
- Integration Capabilities: The tool should seamlessly integrate with your existing workflow and machine learning frameworks.
- Cost-Effectiveness: Evaluate the pricing model to ensure it fits your budget without compromising on essential features.
- Support and Community: A tool backed by a robust support system and active community can enhance your experience tremendously.
Benefits of Using Image Labeling Tools in Business
Implementing these tools into your business processes can yield numerous advantages:
1. Accelerates Project Timelines
The use of image labeling tools significantly shortens the time required to prepare datasets. As projects often hinge on the availability of labeled data, faster data preparation can lead to shorter deployment times for AI and machine learning applications.
2. Improves Model Accuracy
High-quality labeled data leads to the training of more accurate models. Mislabeling can lead to errors, and employing effective tools minimizes this risk by providing clear annotation workflows.
3. Enables Scalability
As businesses grow, so does the need for larger datasets. Image labeling tools facilitate the scaling of data annotation efforts, enabling businesses to keep pace with their evolving requirements.
4. Ensures Compliance and Transparency
In industries like healthcare and finance, maintaining compliance with regulations is crucial. By utilizing robust image labeling tools, businesses can ensure their data practices are transparent and well-documented.
Case Studies: Successful Implementation of Image Labeling Tools
Let’s take a look at how businesses across various sectors have successfully implemented image labeling tools for object detection:
Healthcare Sector
A leading healthcare provider employed an image labeling tool to annotate radiology images. By creating a comprehensive dataset, the organization was able to train models that significantly improved diagnostic accuracy, leading to better patient outcomes.
Automotive Industry
An automotive manufacturer leveraged image labeling for cutting-edge autonomous vehicle technology. By annotating thousands of driving scenario images, the company developed robust models capable of identifying pedestrians, traffic signals, and obstacles, enhancing vehicle safety.
Retail and E-commerce
A major retail brand adopted image labeling tools to automate its product recognition systems. By ensuring accurate labeling of product images, the retailer improved inventory management and customer experience through better search functionalities on its e-commerce platform.
Best Practices for Data Annotation
To maximize the effectiveness of your labeling efforts, consider the following best practices:
- Standardize Annotation Guidelines: Create a comprehensive manual that outlines how to annotate images consistently.
- Train Your Team: Ensure that everyone involved in the annotation process has been trained adequately to follow these guidelines.
- Review and Revise: Regularly review annotated data to identify any patterns of mislabeling and make necessary adjustments.
The Future of Image Labeling Tools
As the landscape of technology evolves, so will the capabilities of image labeling tools. With the integration of advancements such as:
- Automated Labeling: Machine learning techniques will enable automatic labeling, reducing manual effort.
- AI-Powered Suggestions: Tools may offer annotation suggestions based on previous data, further speeding up the process.
- Enhanced User Interfaces: Continuous improvement in UX will make these tools even more accessible.
Conclusion
In the current technological landscape, utilizing an image labeling tool for object detection can provide businesses with a distinct competitive advantage. By implementing these tools, organizations can enhance their operational efficiencies, drive innovation, and significantly improve outcomes across various applications. As you consider incorporating image labeling into your strategy, remember that the quality of your annotated data directly correlates with the success of your machine learning initiatives. Embrace these tools, and unlock the full potential of your data.