The Ultimate Guide to Data Labeling: Tools and Platforms for the Modern Business
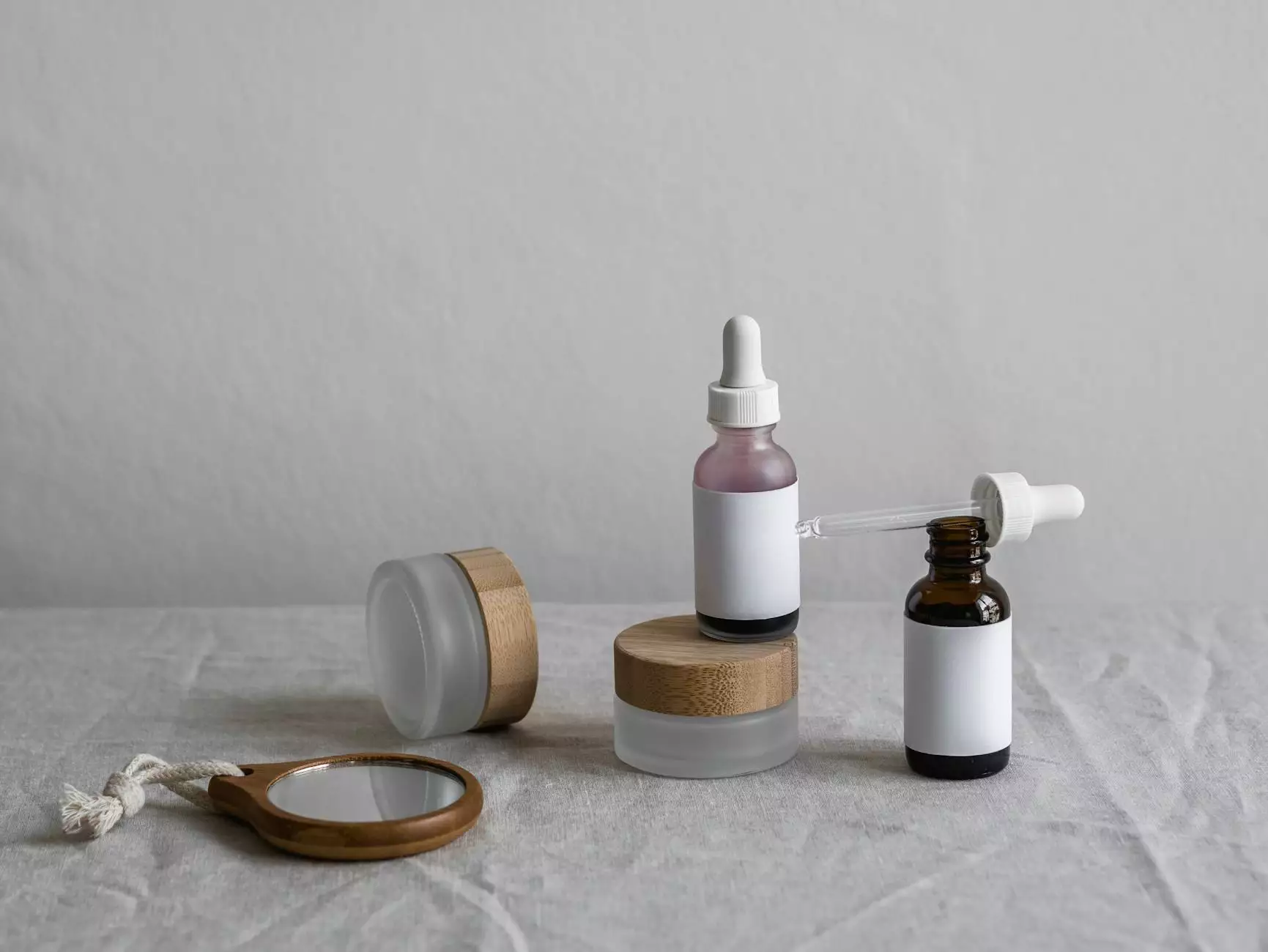
Data labeling has emerged as a pivotal element in the field of artificial intelligence (AI) and machine learning (ML). As businesses leverage these technologies for various applications—from natural language processing to image recognition—the demand for meticulously labeled data is greater than ever. In this comprehensive guide, we will delve into the intricacies of data labeling, explore the essential data annotation tools and platforms, and explain how Keylabs.ai can provide the resources and support you need to excel in your AI projects.
Understanding Data Labeling
Data labeling, at its core, is the process of annotating data to provide meaning to it. It involves identifying and tagging relevant aspects within a dataset. This process is crucial for training machine learning models, as it helps these models learn from examples. Without proper data labeling, AI models can become ineffective or biased, leading to poor decision-making and performance.
The Importance of Data Labeling
The importance of data labeling cannot be overstated. Here are several key reasons why:
- Enhances Model Accuracy: Correctly labeled data helps algorithms learn accurately, leading to improved model performance.
- Facilitates Better Decision Making: With precise data annotations, businesses can extract meaningful insights, fostering informed decision-making.
- Drives Innovation: High-quality labeled datasets enable the development of more sophisticated AI applications, allowing companies to stay ahead of the competition.
- Reduces Risks: By minimizing errors and biases, proper labeling reduces the risk of deploying ineffective AI solutions that could lead to reputational damage.
Types of Data Labeling
Data labeling can be categorized based on the type of data being annotated. Here are the primary types:
1. Image Labeling
Image labeling involves tagging images with relevant labels or classifications. This type of annotation is widely used in applications such as autonomous vehicles, facial recognition, and medical imaging. Common techniques include:
- Bounding Boxes: Drawing rectangles around objects to identify them.
- Segmentation: Dividing images into segments to label specific areas.
- Keypoint Annotation: Marking specific points on objects for tasks like posture detection.
2. Audio Labeling
Audio labeling is essential in speech recognition and music classification. This involves transcribing spoken words into text, identifying sound events, or tagging specific audio features.
3. Text Annotation
In natural language processing (NLP), text annotation includes tasks such as sentiment analysis, named entity recognition, and topic classification. It helps models understand language context and semantics.
Data Annotation Tools: A Closer Look
A variety of tools are available for effectively managing the data labeling process. Here are some of the most prominent data annotation tools currently on the market:
1. Labelbox
Labelbox is a collaborative data annotation platform that enhances workflow management with features such as project tracking and quality assurance. It supports various data types, including images, text, and video.
2. Supervisely
Supervisely is an open-source platform ideal for image labeling and annotations. It offers advanced features like 3D point cloud support and in-built machine learning tools to automate repetitive tasks.
3. VGG Image Annotator (VIA)
This is a lightweight, open-source tool that requires no installation. VIA allows intuitive image annotation and is particularly suitable for researchers and smaller projects.
4. Keylabs.ai
Keylabs.ai offers a robust data annotation platform that excels in providing tailored solutions for businesses. With an emphasis on high-quality data labeling, Keylabs.ai combines advanced algorithms and skilled human annotators to ensure precision in every project.
Choosing the Right Data Annotation Platform
When selecting a data annotation platform, consider the following criteria:
- Scalability: Ensure the platform can handle increasing data volumes as your business grows.
- Ease of Use: A user-friendly interface can significantly expedite the annotation process.
- Quality Assurance: Look for features that ensure the accuracy and quality of labeled data.
- Supported Data Types: Choose a platform that accommodates all data types relevant to your projects.
Best Practices for Effective Data Labeling
To maximize the effectiveness of your data labeling efforts, consider implementing these best practices:
1. Define Clear Annotation Guidelines
Establishing comprehensive and precise guidelines ensures that annotators understand the expectations, leading to consistent results.
2. Use a Diverse Annotation Team
A diverse team of annotators can help minimize biases in the data labeling process, resulting in a more reliable dataset.
3. Implement Iterative Quality Control
Regularly review and refine the labeled data to catch errors and improve the quality of annotations over time.
4. Leverage Automation Where Appropriate
While human input is crucial, automation tools can speed up the labeling process for repetitive tasks, increasing efficiency.
The Future of Data Labeling
The field of data labeling is rapidly evolving, with innovations in technology driving significant changes. Some of the expected trends include:
1. Increased Use of AI and Machine Learning
Automated annotation tools powered by AI will become increasingly sophisticated, enabling faster and more accurate labeling processes.
2. Real-time Collaboration
As remote work continues to grow, platforms will enhance their collaborative features, allowing teams to work simultaneously on data labeling tasks.
3. Enhanced Data Security
With growing concerns over data privacy, platforms that prioritize security measures will become essential for businesses handling sensitive data.
Conclusion
In conclusion, data labeling is a fundamental component of successful AI and ML projects. Understanding the nuances of the labeling process and choosing the right tools and platforms can significantly impact your business's ability to harness the power of data. Leveraging solutions from Keylabs.ai not only streamlines your data annotation workflow but also ensures high-quality labeled datasets that drive innovative AI applications. To thrive in today's data-driven landscape, investing in a robust data labeling strategy is not just advisable—it’s imperative.